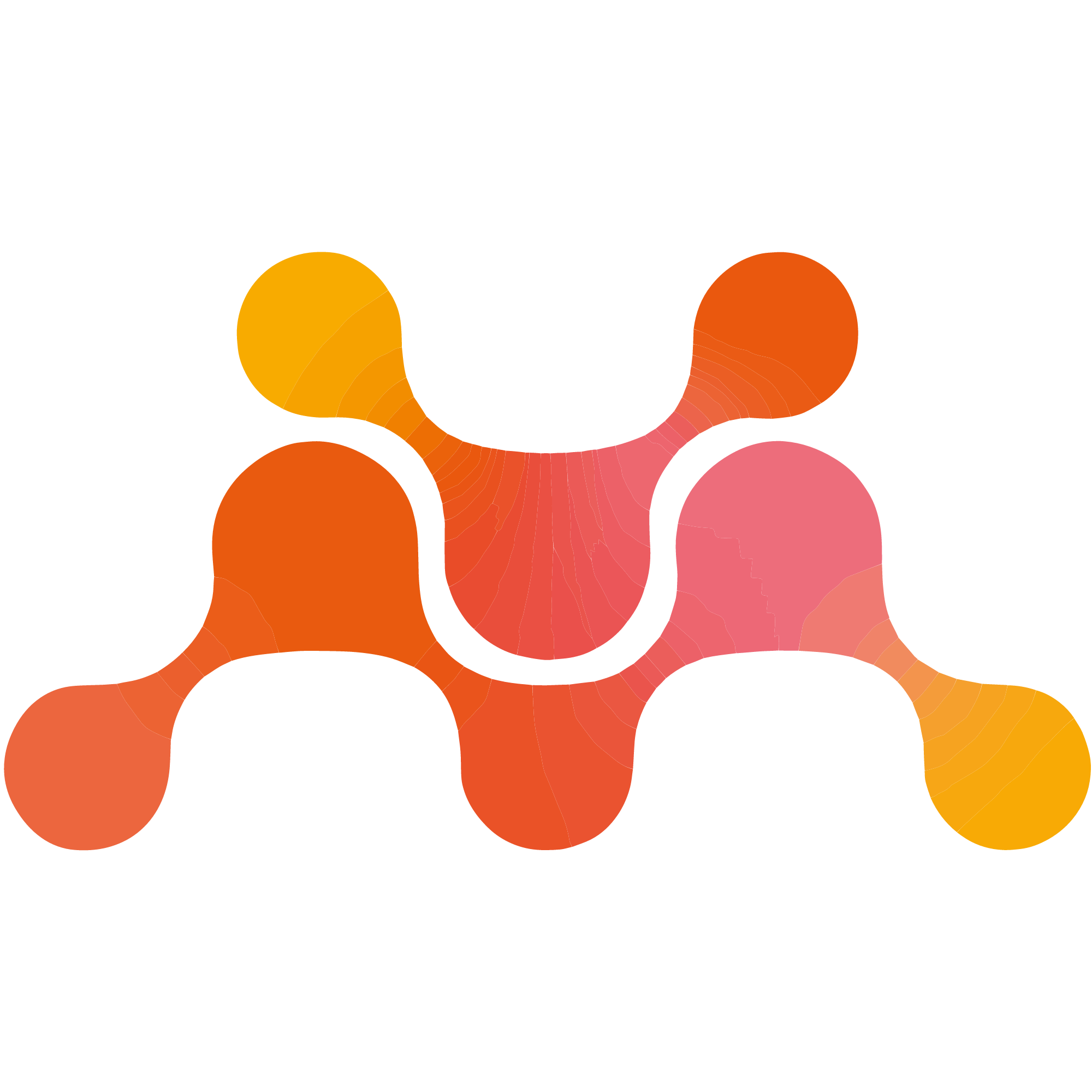
Integration: mixedbread ai
Use mixedbread's models as well as top open-source models in seconds
Table of Contents
Overview
mixedbread ai is an AI start-up that provides open-source, as well as, in-house embedding and reranking models. You can choose from various foundation models to find the one best suited for your use case. More information can be found on the documentation page.
Installation
Install the mixedbread ai integration with a simple pip command:
pip install mixedbread-ai-haystack
Usage
This integration comes with 3 components:
For documents you can use MixedbreadAIDocumentEmbedder
and for queries you can use MixedbreadAITextEmbedder
. Once you’ve selected the component for your specific use case, initialize the component with the model
and the
api_key
. You can also set the environment variable MXBAI_API_KEY
instead of passing the api key as an argument.
Embedders In a Pipeline
from haystack import Document, Pipeline
from haystack.document_stores.in_memory import InMemoryDocumentStore
from haystack.components.writers import DocumentWriter
from haystack.components.retrievers.in_memory import InMemoryEmbeddingRetriever
from mixedbread_ai_haystack.embedders import MixedbreadAIDocumentEmbedder, MixedbreadAITextEmbedder
# Set-up the Document Store and Documents
document_store = InMemoryDocumentStore(embedding_similarity_function="cosine")
documents = [
Document(content="china is the most populous country in the world."),
Document(content="india is the second most populous country in the world."),
Document(content="united states is the third most populous country in the world.")
]
# Indexing Pipeline
indexing_pipeline = Pipeline()
indexing_pipeline.add_component("doc_embedder", MixedbreadAIDocumentEmbedder(model="mixedbread-ai/mxbai-embed-large-v1"))
indexing_pipeline.add_component("writer", DocumentWriter(document_store=document_store))
indexing_pipeline.connect("doc_embedder", "writer")
indexing_pipeline.run({"doc_embedder": {"documents": documents}})
# Query Pipeline
query_pipeline = Pipeline()
query_pipeline.add_component("text_embedder", MixedbreadAITextEmbedder(model="mixedbread-ai/mxbai-embed-large-v1"))
query_pipeline.add_component("retriever", InMemoryEmbeddingRetriever(document_store=document_store))
query_pipeline.connect("text_embedder.embedding", "retriever.query_embedding")
results = query_pipeline.run({"text_embedder": {"text": "Which country has the biggest population?"}})
top_document = results["retriever"]["documents"][0].content
print(top_document)
Reranker In a Pipeline
from haystack import Document, Pipeline
from haystack.document_stores.in_memory import InMemoryDocumentStore
from haystack.components.retrievers.in_memory import InMemoryBM25Retriever
from mixedbread_ai_haystack.rerankers import MixedbreadAIReranker
# Set-up the Document Store and Documents
documents = [
Document(content="china is the most populous country in the world."),
Document(content="india is the second most populous country in the world."),
Document(content="united states is the third most populous country in the world.")
]
document_store = InMemoryDocumentStore()
document_store.write_documents(documents)
# Define the Retriever and Reranker
retriever = InMemoryBM25Retriever(document_store=document_store)
reranker = MixedbreadAIReranker(model="mixedbread-ai/mxbai-rerank-large-v1", top_k=3)
# Rerank Pipeline
rerank_pipeline = Pipeline()
rerank_pipeline.add_component("retriever", retriever)
rerank_pipeline.add_component("reranker", reranker)
rerank_pipeline.connect("retriever.documents", "reranker.documents")
# Query and Rerank
query = "Which country has the second largest population"
results = rerank_pipeline.run({"retriever": {"query": query}, "reranker": {"query": query, "top_k": 3}})
print(results)
Full Example With Metadata
import os
from datasets import load_dataset
from haystack import Pipeline, Document
from haystack.document_stores.in_memory import InMemoryDocumentStore
from haystack.components.writers import DocumentWriter
from haystack.components.retrievers.in_memory import InMemoryEmbeddingRetriever
from mixedbread_ai_haystack import MixedbreadAIDocumentEmbedder, MixedbreadAITextEmbedder, MixedbreadAIReranker
# Set API Key
os.environ["MXBAI_API_KEY"] = "YOUR_API_KEY"
# Load the Dataset and Prepare Documents
ds = load_dataset("rajuptvs/ecommerce_products_clip")
documents = [
Document(
id=str(i),
content=data["Description"], meta={
"name": data["Product_name"],
"price": data["Price"],
"colors": data["colors"],
"pattern": data["Pattern"],
"extra": data["Other Details"]
}) for i, data in enumerate(ds["train"])
]
meta_fields = documents[0].meta.keys()
# Define the Components
document_store = InMemoryDocumentStore(embedding_similarity_function="cosine")
document_writer = DocumentWriter(document_store=document_store)
embedding_retriever = InMemoryEmbeddingRetriever(document_store=document_store, top_k=20)
embed_model = "mixedbread-ai/mxbai-embed-large-v1"
reranking_model = "mixedbread-ai/mxbai-rerank-large-v1"
text_embedder = MixedbreadAITextEmbedder(model=embed_model)
document_embedder = MixedbreadAIDocumentEmbedder(model=embed_model, max_concurrency=3, meta_fields_to_embed=meta_fields, show_progress_bar=True)
reranker = MixedbreadAIReranker(model=reranking_model, meta_fields_to_rank=meta_fields, top_k=5)
# Indexing Pipeline
indexing_pipeline = Pipeline()
indexing_pipeline.add_component(instance=document_embedder, name="document_embedder")
indexing_pipeline.add_component(instance=document_writer, name="document_writer")
indexing_pipeline.connect("document_embedder", "document_writer")
# Query Pipeline
query_pipeline = Pipeline()
query_pipeline.add_component(instance=text_embedder, name="text_embedder")
query_pipeline.add_component(instance=embedding_retriever, name="embedding_retriever")
query_pipeline.add_component(instance=reranker, name="reranker")
query_pipeline.connect("text_embedder", "embedding_retriever")
query_pipeline.connect("embedding_retriever.documents", "reranker.documents")
# Index the dataset
indexing_pipeline.run({"document_embedder": {"documents": documents}})
# Query to get results
query = "I am looking for a regular fit t-shirt in blue color. Ideally without any prints. What are my options?"
results = query_pipeline.run(
{
"text_embedder": {"text": query},
"reranker": {"query": query}
}
)
print(results["reranker"]["documents"])